Recently, the international Visual Object Tracking Challenge 2022 (VOT2022) held the award ceremony and competition summary during ECCV2022 (the European International Conference on Computer Vision). The IIAU Laboratory led by Professor Lu Huchuan from the Department of Electronic Information and Electrical Engineering of Dalian University of Technology won the championship in three courses, namely long time, depth and RGBD. Since 2018, the IIAU Lab has won several courses in the international competition for five consecutive years. The champion algorithm VITKT_M of the long course was jointly completed by doctoral students Zhao Jie, Chen Xin and Liu Chang, supervised by Professors Lu Huchuan, Wang Dong and Peng Houwen. MixFormerD/MixFormerRGBD, the champion algorithm of Deep Track and RGBD track, was jointly completed by doctoral student Lai Simiao, master student Li Ming and doctoral student Zhu Jiawen. The instructors were Professor Lu Huchuan, Associate Professor Wang Lijun and Professor Wang Dong. The first authors, Zhao Jie and Lai Simiao, were invited to give a tele-conference report at the award ceremony respectively to introduce the core content of their winning algorithms to the participants.
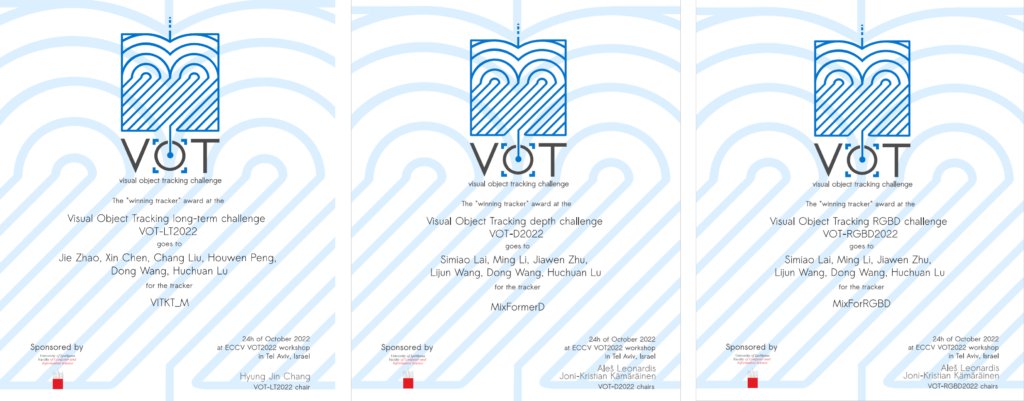
The Visual-Object-Tracking Challenge (VOT) is the world's most authoritative online target Tracking Challenge. Every year, the VOT workshop is held during the top conference to measure the performance of the single target tracking algorithm in complex scenarios. The VOT contest is also considered to be the most difficult in the field of visual tracking, far surpassing other data sets, due to the fact that the evaluation sequence is updated every year and the accuracy of the labeling is improved every year. So every year, the best tracking algorithms put their best foot forward, creating a spark of inspiration in the fierce competition. Since 2017, IIAU Laboratory has consistently won the championship in several circuits of the international competition, beating Oxford University, Carnegie Mellon University, Microsoft Research Asia and other internationally renowned AI laboratories and universities. Among them, in VOT2017, doctoral student Sun Chong won the first place in the open group. In VOT2018, Master student Zhang Yunhua won the champion of the long course. VOT2019 by master student Dyke Nan won the long track champion; In VOT2020, master students Dai Keman, Yan Bin and Wang Yingming won the champion of long time, real time and depth respectively. In VOT2021, doctoral students Chen Xin and Zhang Xinyu won the champion of the long course and the deep course respectively. In this year's VOT2022, doctoral students Zhao Jie and Lai Simiao won the three courses: long time, depth and RGBD.
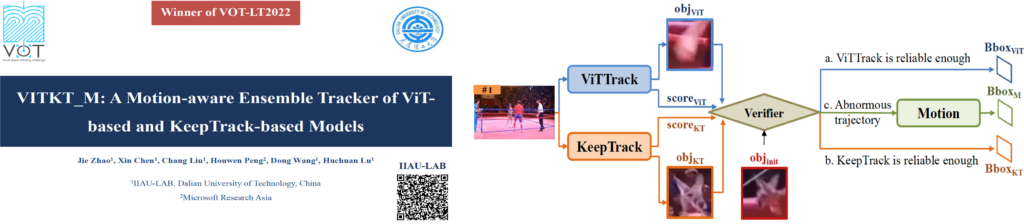
The frequent disappearance and reappearance of targets is a major challenge for long-term tracking tasks. Compared with the short-time tracker, the long-time tracker needs to have the ability to judge whether the target disappears or not and quickly recover the target. In addition, target disruptors are another challenge to tracking robustness. This time, IIAU team proposed a motion sensing integrated tracking algorithm VITKT_M, which won the champion of the long track. Specifically, two tracking models with complementary attributes are integrated through the validator, the optimal results are selected, and a motion module is proposed to detect the abnormal trajectory of the target, and then the drift of the tracker is constrained. The complementary tracking models are ViTTrack model based on Transformer and KeepTrack model for distractor challenge. The validator mainly selects and judges based on the confidence score corresponding to the tracking model and the additional similarity estimation.
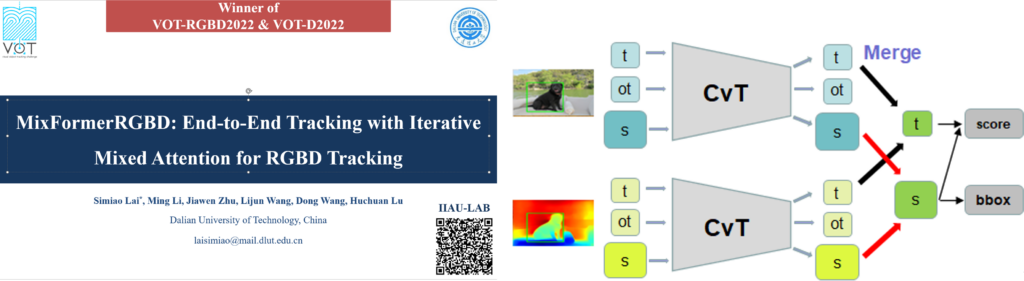
For RGBD tracking, it is an urgent problem to be solved how to effectively integrate depth features to make the tracker more robust in complex scenes that ordinary RGB trackers can't cope with well, such as illumination variation, analogue interference and field group set. The champion algorithms of the previous two years only used depth information to heuristically judge whether the target was blocked or out of view. This year, with the emergence of tracking training set with depth and the short setting of VOTRGBD2022 track, the team proposed an end-to-end tracking algorithm MixFormerRGBD based on interactive mixed attention for RGBD tracking. The algorithm adds a depth map branch based on MixFormer to receive the depth map encoded in JET style. After the interactive mixed attention backbone network, the RGB and depth search features injected with the target information are respectively obtained, which are fused by the operation of taking the maximum value of each element and then sent to the subsequent coordinate regression and target score prediction network. On the basis of loading pre-training RGB parameters, the team used the DepthTrack training set and the depth map generated by DenseDepth on LaSOT, GOT10k and COCO to fine-tune the overall network.
Similarly, for the depth tracking track, as only the depth map can be utilized, the team replaced the RGB input of MixFormerRGBD and replaced it with the normalized depth map for fine tuning, and proposed the tracking drift penalty strategy to punish the untrusted displacement mutation in the tracking process. Finally achieved a big lead on the depth and RGBD track. As you can see below, we are 1.7 percentage points ahead of the second place on the VOT-RGBD2022 circuit and 2.7 percentage points ahead of the second place on the VOT-D2022 circuit.
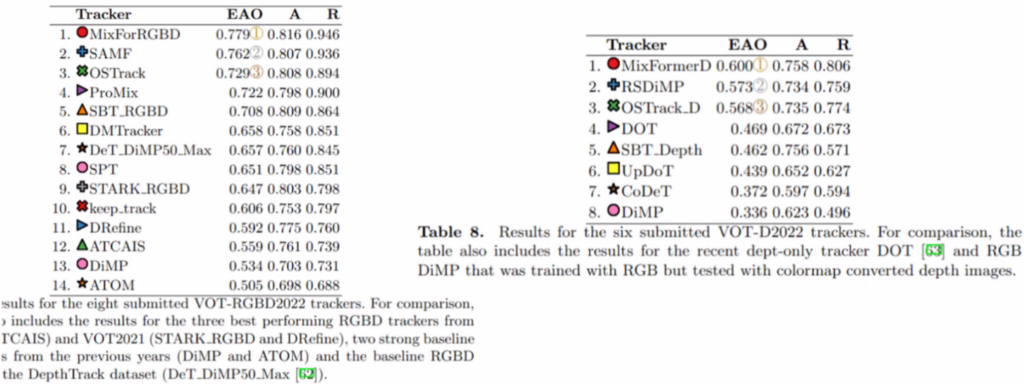